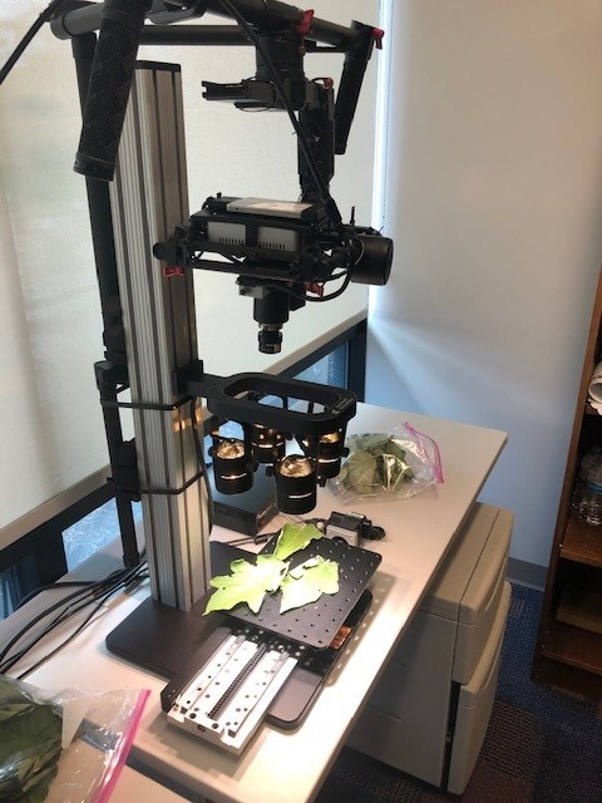
By Yiannis Ampatzidis and Pamela Roberts
Accurate and rapid disease identification at the beginning of an outbreak is essential for implementing effective management tactics. Diagnosis based on visual symptoms is often compromised by the inability to differentiate among similar symptoms caused by plant pathogens and abiotic disorders.
Recent technological advances in sensors, machine vision, mechatronics, big data analytics and artificial intelligence (AI) have enabled the development and implementation of remote sensing technologies for rapid disease identification and management. Early disease-detection technologies can determine the spatial distribution of a disease outbreak for targeting precise management tactics.
These technologies can be used to distinguish between a variety of disease symptoms in the field and/or transplant house, thus reducing both time and cost for diagnosis and management. This article presents examples of recent advances and the latest technical developments in machine learning, which is an application of AI, for intelligent plant protection.
Machine Learning
The simulation of human intelligence processes by machines (e.g., computers) is known as machine learning (ML). It creates models based on sample data, referred to as “training data,” to make predictions or judgments without being explicitly programmed.
ML typically involves three main steps: learning, testing and self-adjusting. ML models try to mimic the step-by-step reasoning that humans employ when solving problems or making logical inferences. For example, artificial neural networks, an ML methodology, are inspired by our understanding of the biology of our brains, mimicking the vast number of interconnected neurons.
Identification Via Images
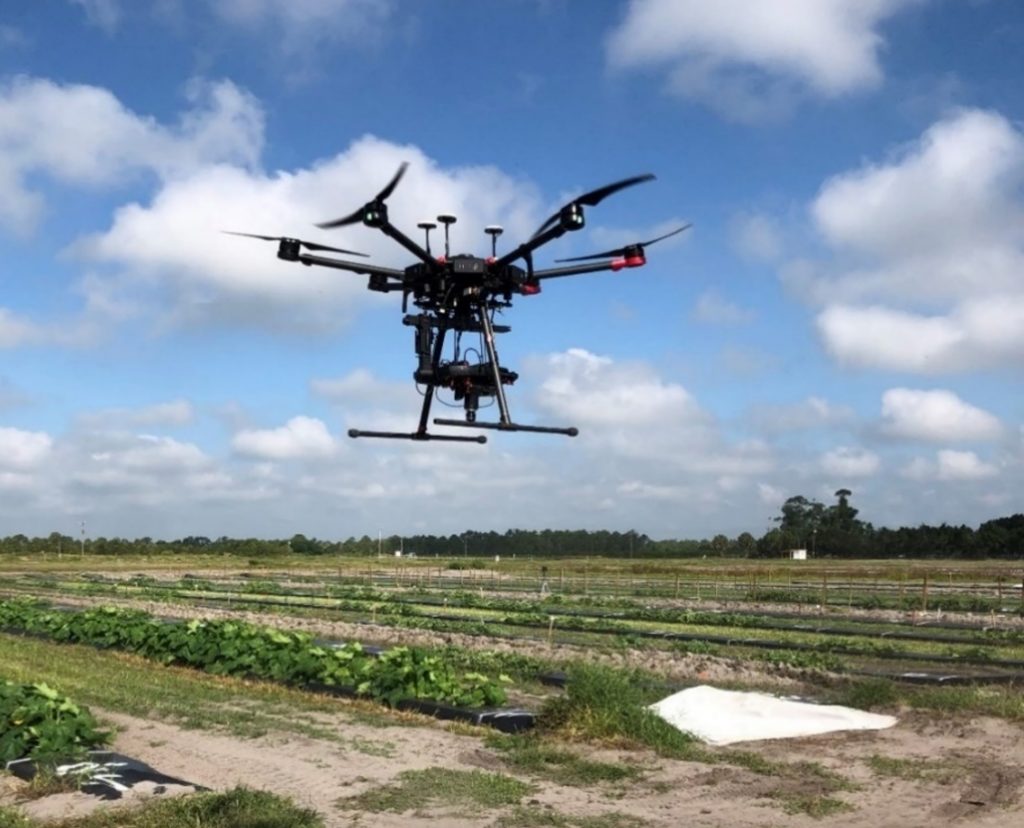
Disease identification in the field can be a very complicated procedure because it needs experienced personnel and frequent monitoring. Currently, growers use mainly visual observations to identify diseases in vegetable fields. The goal is early disease detection, so that growers can apply the right treatment(s) and control the spread.
Recently, several image-based pattern-recognition systems have been developed for pest and disease detection. Some of these systems utilize ML models to detect a pest or visual symptoms of a disease from images. By evaluating a vast amount of training data, an ML-based image recognition technology can learn to identify and characterize objects (e.g., symptoms of a specific disease) in pictures.
With these systems, users can take photos of a possibly infected plant, upload the images from their mobile device or computer, have the images processed remotely through the cloud by an ML model and receive a prompt diagnosis of the specimen. Several software options (e.g., mobile or web applications) are commercially available for image- and symptom-based disease detection. However, identification of diseases by pictures alone relies upon the quality of the photos and typical diagnostic symptoms or signs of the pathogen.
Early Detection
Early identification of a specific disease in a crop helps growers make the right decisions in selecting appropriate management practices. An infected plant may exhibit similar visual symptoms despite being caused by different pathogens. This is especially the case in early disease development stages, which makes it difficult to identify and differentiate between the diseases by visual observations.
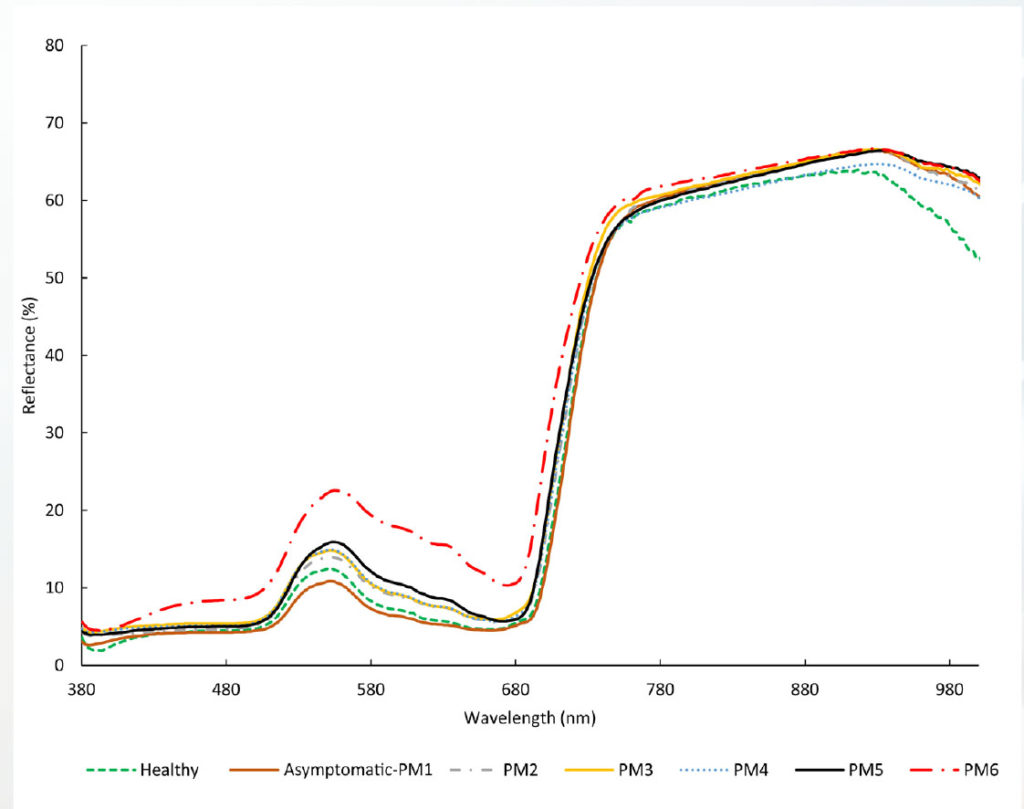
For example, early lesions of target spot (TS) caused by the fungus Corynespora cassiicola and bacterial spot (BS) caused by Xanthomonas perforans may be similar in tomato plants. Any delay or incorrect diagnosis can lead to improper management decisions that could potentially increase the spread within the field, reduce crop health and yield, and increase economic loss. Many diseases need only a few days to spread rapidly in the field.
For early plant disease and stress identification, multispectral and hyperspectral sensing and ML have been utilized with promising results. These sensors measure the energy a surface (e.g., leaf or plant canopy) reflects at a specific wavelength. Any change that might occur and disturb the plant canopy or the leaf surface (e.g., caused by a disease) would affect the light reflectance and diffuse the light direction. By detecting these changes, it is possible to identify abnormalities in plants. This technology has the potential to detect and distinguish a specific disease in an asymptomatic stage (before symptoms become obvious to direct visual observations).
One example is a study at the University of Florida Institute of Food and Agricultural Sciences (UF/IFAS) Southwest Florida Research and Education Center (SWFREC). The study utilized a hyperspectral sensing system and novel ML techniques for early detection and classification of three critical tomato diseases (BS, TS and tomato yellow leaf curl virus) in the laboratory and field with high accuracies (more than 90%). In the laboratory, a benchtop hyperspectral imaging system (Figure 1) was used. In the field, an unmanned aerial vehicle equipped with the same hyperspectral sensor (Figure 2) was utilized, and ML models were developed to classify diseases in early (asymptomatic) and late (symptomatic) development stages.
In another SWFREC study, hyperspectral imaging and ML were utilized to develop a technique for detecting different disease development stages (asymptomatic, early, intermediate and late) of powdery mildew (PM) in squash, in the laboratory and field. Powdery mildew is a common disease on squash in the United States that can decrease yield and reduce fruit quality if it is not controlled early. First, the spectral signatures of healthy and PM-infected plants were created (Figure 3). Then, ML models were utilized to classify the disease development stages. As expected, the higher classification accuracy was achieved in the late stage (~97%), and the lower accuracy (~84%) in the early disease-detection stages.
Both studies identified the most significant wavelengths for disease detection and classification to develop low-cost (multispectral) sensing systems for rapid disease detection in the field or transplant house. Additional studies are planned to evaluate the detection accuracy of the proposed techniques in commercial fields.
Yiannis Ampatzidis is an associate professor and Pamela Roberts is a professor, both at the UF/IFAS SWFREC in Immokalee.